Derived Demand Forecasting in Agriculture Explained
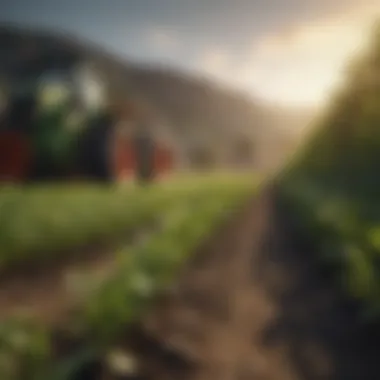
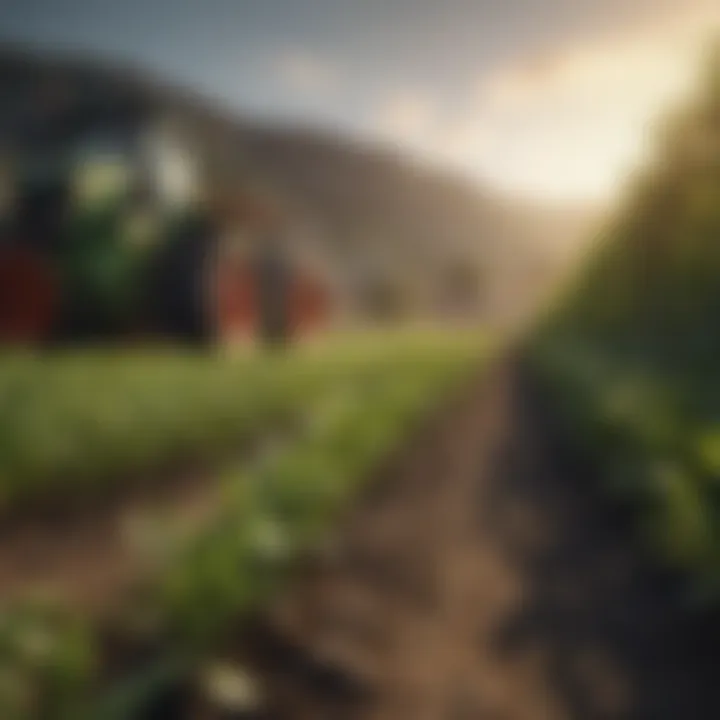
Intro
The landscape of agriculture is continually shifting, influenced by various factors like climate change, market demands, and technological advancements. One of the forecasting techniques that has gained traction is the derived demand method. Understanding derived demand is akin to getting to the root of what drives agricultural production. It connects the dots between consumer preferences for food products and the requirements of farmers to produce those goods efficiently. This article aims to dissect the derived demand method, illustrating its significance in the agricultural sector and how it can refine decision-making processes for farmers and economists alike.
Latest Trends in Agriculture
Overview of Current Trends
As we look into the realm of modern agriculture, several trends emerge that are reshaping how products are cultivated, marketed, and consumed. For instance, there has been an increasing tilt towards organic farming, not just as a buzzword but as a response to the rising consumer demand for healthier, pesticide-free options. This trend cascades into derived demand forecasting; as more consumers opt for organic, farmers must adjust what and how they grow.
Furthermore, regenerative agriculture is on the rise, focusing on restoring soil health and increasing biodiversity. This practice not only boosts yields in the long run but also aligns with the growing consumer interest in sustainability. Farming practitioners need to be cognizant of such movements, as they would undoubtedly affect market demand, thus impacting their strategies and operations.
Impact of Technology on Farming Practices
The agricultural field is no stranger to technological innovation. Precision agriculture, for example, utilizes GPS and IoT devices to gather essential data. Farmers can monitor soil conditions, crop health, and weather patterns in real-time. These advancements transform the landscape and provide farmers with critical information needed to make informed decisions, which in turn relates to derived demand forecasting. As farmers can predict the supply needed to meet consumer demand more accurately, they can optimize their operations accordingly.
"Technology in farming is not just about machinery; it's about understanding the flow of supply and demand, making informed decisions in light of data-driven insights."
Sustainable Practices: Towards a Greener Future
Importance of Sustainability in Agriculture
The urgency of addressing climate change cannot be overstated. Sustainable practices in agriculture are essential for ensuring the long-term viability of farming. Incorporating derived demand methods allows for a more robust understanding of how sustainability impacts consumer choices. When consumers lean towards products that are sustainably farmed, it shifts the demand curve, prompting farmers to adapt their practices accordingly.
Methods for Sustainable Farming
Several methods are gaining traction in sustainable farming. Here are some noteworthy techniques:
- Crop rotation: This method helps in maintaining soil health, managing pests, and enhancing biodiversity.
- Agroforestry: Integrating trees and shrubs into crops and livestock systems helps restore ecosystems while providing additional yield.
- Water conservation techniques: Methods like drip irrigation optimize water usage, crucial in many regions facing drought.
Farmers employing these methods not only contribute to environmental protection but also position themselves to meet the rising consumer desire for sustainable products.
Gardening Techniques and Tips
Essential Gardening Tools and Equipment
For those who engage in small-scale farming or gardening, having the right tools is paramount. Essential tools can include:
- Trowels: Handy for planting and soil manipulation.
- Pruners: Useful for trimming and shaping plants for better growth.
- Rakes: Necessary for spreading mulch or preparing soil.
Employing the right tools can enhance efficiency and productivity in gardening practices.
Seasonal Gardening Practices
Timing is often an unsung hero in the gardening world. Understanding seasonal challenges and advantages can dramatically affect yield. For example:
- In spring, focus should be on planting cool-season crops like lettuce and peas.
- Summer often calls for heat-loving varieties such as tomatoes and peppers.
By aligning gardening practices with seasonal changes, both professional farmers and hobbyists can optimize their output while understanding the underlying demand that drives their efforts.
Prelude to Derived Demand
Understanding the concept of derived demand is crucial for grasping how agricultural markets operate. Derived demand refers to the demand for goods or services that is influenced by the demand for another good. In agriculture, this often means that the demand for products such as crops or livestock is contingent on the needs of consumers or industries that utilize these agricultural outputs. This interdependence shapes not just market behaviors, but also forecasting methods aimed at predicting future needs in the sector.
Definition and Concept
Derived demand in agriculture cannot be merely confined to theoretical discussions. At its core, it represents a fundamental relationship between agricultural products and the consumer market. When a farmer considers which crops to plant, decisions are often rooted in external demands—like the preferences of restaurants, grocery stores, or international buyers. For instance, if there’s a surge in popularity for organic soil, demand for organically produced vegetables and fruits skyrockets as traders anticipate higher returns for such goods. Thus, the concept of derived demand becomes a strategic tool, aiding farmers in making informed choices that align with market trends—avoiding the pitfalls of planting crops that might not sell well.
The derived demand phenomenon is not just confined to crop selection but spans the entire supply chain of agricultural production. For example, increased interest in plant-based diets has coincided with a rise in demand for legumes and grains, prompting farmers to pivot their cultivation focus accordingly. If consumers suddenly develop a taste for quinoa, suddenly, the agricultural landscape changes, pushing local farmers to reevaluate their planting decisions.
Importance in Economic Theory
In the grand scheme of economic theory, derived demand serves as a bridge between consumer behavior and agricultural production. It integrates substantial aspects of supply-demand mechanics into a cohesive framework that informs various stakeholders in the agricultural sector. Economic models that include derived demand offer a clearer picture of market dynamics. They allow for predictive capabilities when understanding shifts in consumer preferences.
Utilizing derived demand appropriately can lead to beneficial outcomes for both farmers and consumers. For instance:
- Pricing: If farmers recognize that there’s upsurging demand for a particular fruit, they might adjust their pricing strategies to maximize profits.
- Resource allocation: Knowing which crops are in higher demand helps farmers allocate resources effectively, ensuring that labor, land, and capital are used efficiently.
- Policy-making: Understanding how derived demand plays into market predictions can inform policymakers. This can lead to regulations that promote sustainable agricultural practices and stabilize food prices for consumers.
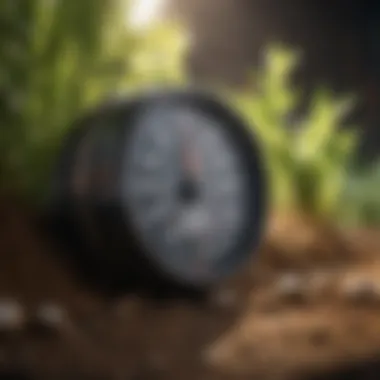
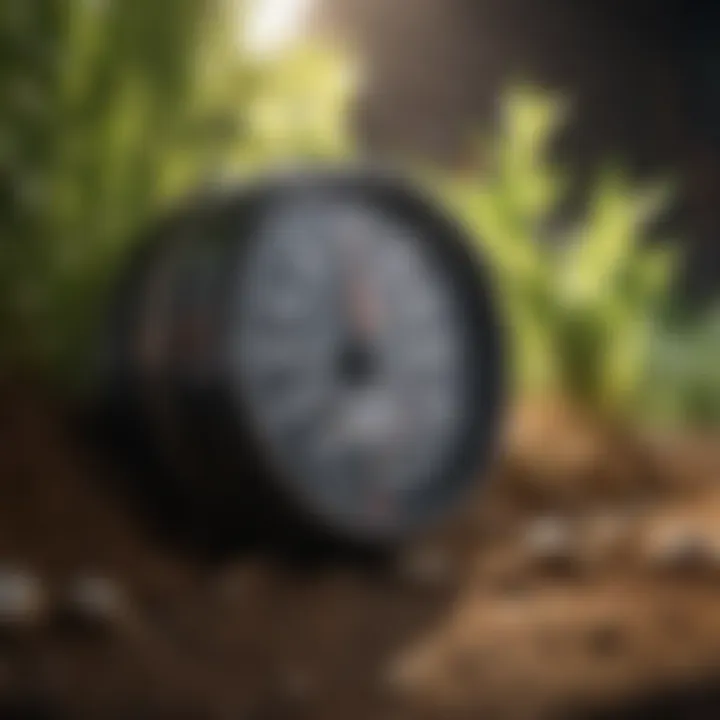
Essentially, derived demand sheds light on the symmetric relationship between what consumers show interest in and what producers need to provide. It’s not merely about immediate supply; it encompasses the entire economic stimuli that shape production decisions in agriculture. As the landscape of agriculture continues to evolve, understanding derived demand has become indispensable for stakeholders aiming to refine their strategies and solutions, positioning them favorably amid unpredictable market fluctuations.
“The effects of consumer behavior on agricultural production cannot be understated; it’s the very heartbeat of market strategy.”
This exploration into derived demand not only clarifies its definition and importance in economic theory but lays a foundational understanding for further discussions surrounding forecasting methods in agriculture.
The Derived Demand Method in Forecasting
The derived demand method of forecasting holds a significant place in agricultural predictions, as it connects consumer needs to the agricultural output needed to satisfy those needs. Understanding this relationship is crucial for farmers, agronomists, and agricultural economists aiming to optimize production and allocate resources efficiently. By focusing on how shifts in consumer demand affect agricultural outputs, stakeholders can make more informed decisions, which ultimately enhance productivity and profitability in the sector.
Overview of Forecasting Techniques
When it comes to forecasting in agriculture, various techniques have risen to prominence. Each method has its strengths, depending on the specific context and data availability. Here are a few notable techniques:
- Qualitative Forecasting: This method relies heavily on expert opinions, market research, and informal discussions. While it can yield valuable insights, its subjective nature may introduce bias.
- Quantitative Forecasting: A more data-driven approach, this technique utilizes mathematical models and historical data to predict future trends. For instance, linear regression models can estimate demand based on past sales data, making it easier to project future needs using statistical analysis.
- Time Series Analysis: This method analyzes data points collected or recorded at specific time intervals. It helps in identifying patterns, seasonality, and trends that emerge over time, allowing stakeholders to anticipate demand peaks and troughs.
- Causal Models: These models consider causal relationships between different variables, such as the impact of weather patterns on crop yields. Incorporating variables like economic indicators or technological advancements can enhance forecasting accuracy.
The selection of a forecasting technique should be tailored to the specific agricultural context, considering factors like data availability, market conditions, and the nature of the products being forecast.
How Derived Demand Influences Forecasting
Derived demand intricately influences forecasting by connecting consumer behavior to agricultural production needs. Essentially, an increase in demand for a particular food item leads to a corresponding increase in demand for the inputs needed to produce that item. This dynamic shapes forecasting efforts:
- Shifting Preferences: Changes in consumer preferences, such as a rising trend for organic produce, can drastically alter the required crop mixes. Understanding these shifts can help farmers adjust their planting strategies accordingly.
- Price Elasticity: The responsiveness of demand to price changes is crucial in forecasting. If consumers are highly sensitive to price hikes, forecasting must account for possible decreases in demand for higher-priced crops.
- Economic Indicators: Broader economic trends, like inflation or employment rates, also factor into derived demand. Higher employment often translates to increased disposable income, which supports greater spending on diverse agricultural products.
- Seasonality: Demand for certain crops is heavily seasonal, influenced by cultural practices and consumer habits. Forecasting must take these cycles into account, accurately reflecting periods of high and low demand.
Incorporating the concept of derived demand into forecasting provides a more nuanced view of market dynamics. Farmers and stakeholders who grasp these relationships can better position themselves in an increasingly competitive agricultural landscape. By proactively adapting to forecasted changes, they can optimize their resource allocation and investment decisions, paving the way for sustainable growth and profitability.
"Understanding derived demand is like having a compass in the complex world of agriculture; it guides decisions that can lead to success or failure."
Key Factors Driving Derived Demand
Derived demand in agriculture doesn’t exist in a vacuum; it is fundamentally shaped by various-key factors that interplay in complex ways. Understanding these factors is crucial for accurate forecasting and effective decision-making by stakeholders in the agricultural sector. This section explores three pivotal elements: consumer preferences and trends, economic indicators, and technological advancements, each influencing the derived demand and consequently the agricultural landscape.
Consumer Preferences and Trends
In today’s fast-paced world, consumer preferences can shift almost overnight. The agricultural sector must be nimble, staying in tune with what buyers want. A perfect illustration of this is the growing appetite for organic produce. As individuals become more health-conscious, they increasingly seek out products free from pesticides and other chemicals.
This trend raises the demand for specific crops, encouraging farmers to pivot their strategies accordingly. If we examine the changes in dietary habits over the last decade, it becomes evident that factors like sustainability, ethical farming practices, and even local sourcing are driving the choices consumers make.
- Trends to note:
- Health consciousness leads to a greater demand for organic fruits and vegetables.
- Growing interest in plant-based diets increases the demand for certain crops.
- Seasonal trends can affect demand, urging producers to adapt accordingly.
A farmer who keeps abreast of these changes can optimize their crop selection, ensuring they do not end up with a surplus of unwanted products. Ultimately, carefully studying these shifting preferences helps in crafting effective marketing strategies while reinforcing the importance of market research.
Economic Indicators
Economic indicators serve as signposts guiding firms on the path of derived demand. They provide vital information regarding consumer spending, inflation rates, and overall economic health, all intersections where demand lives. An uptick in disposable income suggests consumers may be willing to spend more on premium agricultural products, driving demand in certain categories.
Conversely, during economic downturns, demand usually contracts. Look at the recent fluctuations in commodity prices; they can tell us much about future demand trends.
Key indicators to monitor include:
- Gross Domestic Product (GDP): A rising GDP often correlates with increased demand for a variety of agricultural goods.
- Consumer Price Index (CPI): Changes in this index affect purchasing power, impacting demand levels.
- Unemployment Rates: High unemployment often translates into reduced consumer spending, affecting demand.
Understanding these economic indicators allows farmers and agribusinesses to anticipate potential risks and make informed decisions regarding production levels and resource allocation.
Technological Advancements
Technological advancements in agriculture are not just about fancy gadgets – they redefine the foundational practices of the industry. From precision farming to automated systems, technological innovations allow farmers to be more efficient and responsive to derived demand.
Imagine employing drones to monitor crop health or utilizing software that analyzes soil conditions in real-time. These advancements can lead to more informed decisions regarding what to plant and when, ultimately influencing the derived demand for certain crops.
- New technologies to consider:
- Artificial Intelligence: Enhances predictive capabilities in commodity forecasts.
- Genetic Engineering: Makes it possible to breed crops that can withstand adverse conditions, increasing yield and meeting market demands more effectively.
- Blockchain Technology: Offers traceability in the supply chain, appealing to consumers increasingly concerned about the origins of their food.
Applications of Derived Demand Method in Agriculture
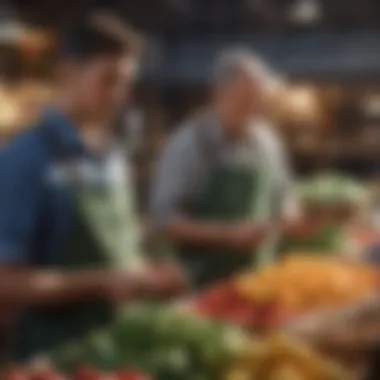
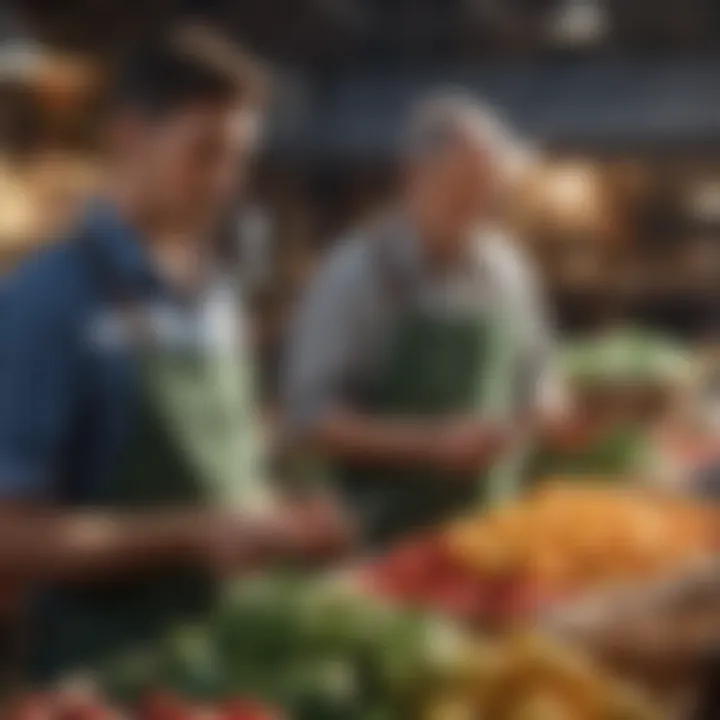
Derived demand forecasting is a crucial area of focus in agriculture, providing insights on how various factors influence the demand for agricultural products. With agriculture being so intertwined with economic and social trends, understanding how derived demand plays out can significantly aid stakeholders in making informed choices. By utilizing derived demand, farmers and agri-businesses can better align their strategies with market needs and anticipate fluctuations, thus enhancing both productivity and profitability.
Crop Demand Forecasting
When we talk about crop demand forecasting, we must consider multiple angles. Derived demand in crops arises from the consumer's desire for food products. For instance, if there’s a surge in demand for plant-based diets, crops like soybeans or lentils become more desirable. Farmers can use this knowledge to plant accordingly, ensuring they meet market demand and avoid losses from excess supply.
Understanding this trend can mean the difference between a farmer's success or failure. Timely data collection and analysis can lead farmers to adjust their crop choices based on consumer behavior, seasonal trends, and economic factors. The information enables farmers to focus on high-demand crops, tailoring planting schedules and resource allocation to maximize output and revenue.
Furthermore, tools like commodity futures markets can help farmers lock in prices, taking advantage of derived demand signals. By forecasting potential shifts, they can hedge against price volatility and demand decline, thus acting proactively rather than reacting to market changes late.
Labor and Resource Allocation
Labor and resource allocation decisions are directly influenced by derived demand forecasts. When yield expectations are high due to increased crop demand, farmers need to ensure they have the right amount of labor and resources at hand. For example, if farmers anticipate dealing with a bumper crop of corn, they have to gear up by hiring additional workers, securing machinery, and managing the supply chain effectively.
However, misjudging demand can lead to underutilization or overextension of resources. It’s a balancing act where precision is key. Compounding this are factors like weather, which can be unpredictable but can severely impact the available labor force and resources. In a situation where a farmer underestimates the need for labor due to low initial demand, they might find themselves scrambling to harvest at peak times, risking loss from overripe crops. By forecasting and adjusting labor inputs based on anticipated demand, farmers can efficiently allocate their resources, optimizing productivity and minimizing waste.
Investment Decisions in Agribusiness
Investment decisions are pivotal in the context of derived demand forecasting. As farmers and agribusinesses seek to expand their operations or upgrade existing equipment, a solid understanding of future demand is essential. A farmer considering investing in a new irrigation system will weigh the potential returns based on projected crop yields and consumer preferences.
In this regard, data-driven decisions matter. A farmer might look at statistics showing an escalation in demand for organic produce and decide to pivot their investments accordingly. Alternatively, shifts in consumer preferences toward sustainability can influence investment in technologies that support organic farming or reduce environmental impact.
Moreover, funding for research and development in new agricultural technologies often depends on forecasting derived demand. If there's an apparent increase in demand for bioengineered crops that resist pests and drought, investors are more likely to back innovations in that area. Conversely, investments in sectors with dwindling demand could lead to wasted resources. Therefore, proximate and future demand insights are vital for driving strategic investment decisions in agribusiness.
Understanding and acting on derived demand in agriculture empowers farmers to navigate complex market landscapes, aligning outputs with consumer needs. It’s not just about planting seeds; it’s about planting the right seeds.
Methodological Approaches to Derived Demand Forecasting
Understanding methodological approaches to derived demand forecasting holds significant weight in agriculture's ever-evolving landscape. These methods equip farmers, agronomists, and economists with valuable insights to make informed decisions. With agriculture becoming increasingly complex due to market changes, technology, and consumer behavior, reliable forecasting methods are crucial.
Digging deeper, both quantitative and qualitative methodologies offer distinct benefits. The quantitative methods rely heavily on numerical data and statistical techniques, providing a solid foundation for predicting future demand through mathematical models. Meanwhile, qualitative approaches focus more on human factors, subjective insights, and market sentiments that numbers alone can't capture. Together, these methods create a comprehensive toolkit for nuanced decision-making.
Moreover, considering the diverse agricultural environments across the globe means choosing the right methodology can greatly influence outcomes. Not all regions respond to the same forecasting techniques, so understanding local contexts becomes essential.
Quantitative Methods
Quantitative methods in derived demand forecasting revolve around numerical data analysis. Utilizing statistical models, these techniques can predict demand changes based on observable variables.
Some key aspects of quantitative methods include:
- Time Series Analysis: This technique involves analyzing historical data to identify patterns or trends over time, allowing forecasts that can adapt to expected future behaviors.
- Econometric Modeling: Think of it as a bridge between economic theory and real-world data. It uses economic models to represent how different variables interact, helping predict demand based on several factors, such as price changes or cost inputs.
- Regression Analysis: This statistical method helps establish whether, and how strongly, different variables are related. For instance, it can help analyze how a rise in consumer income might impact the demand for organic vegetables.
The reliability of quantitative methods largely lies in the accuracy of the data used. Hence, maintaining data quality and making regular updates is essential for effective forecasting.
Qualitative Approaches
On the flip side, qualitative methods bring the human element into forecasting, focusing on attitudes, beliefs, and experiences that might not be reflected in numerical data. These aspects often end up being crucial in understanding consumer behavior.
Some core components of qualitative approaches are:
- Focus Groups: These small group discussions can reveal deeper insights into consumer preferences or market trends. They allow stakeholders to gather feedback on products and services.
- Expert Interviews: Engaging with experts in specific fields can yield invaluable insights that numbers can’t shine light on. These discussions can expose market sentiments or emerging issues.
- Case Studies: Analyzing specific instances can highlight practical challenges and successes, allowing practitioners to draw parallels to current situations.
Qualitative approaches draw heavily on human dynamics and can provide context to changes that quantitative data cannot account for. While subjective, these methods can uncover unexpected market shifts, helping ensure more rounded and practical predictions.
In summary, embracing both methodological approaches lays a solid foundation for comprehensive derived demand forecasting. By integrating quantitative data's rigor with qualitative insights' richness, stakeholders in agriculture can navigate their paths with greater confidence, ensuring decisions are informed and relevant.
Case Studies in Derived Demand Forecasting
When it comes to understanding how derived demand forecasting operates in the agriculture sector, examining practical case studies can provide invaluable insights. These real-world applications illustrate not only the methodology but also the nuances that come into play when implementing these forecasting techniques. Case studies often highlight the intricacies of data collection, analysis, and the eventual impact on decision-making for various stakeholders involved in agriculture.
Successful Implementations across Regions
Several regions around the world have seen successful applications of derived demand forecasting in agriculture. One notable example can be found in the Midwest of the United States, where farmers adopted this forecasting method to better anticipate demand for corn and soybeans. By analyzing consumer preferences and market trends, they adjusted planting strategies accordingly. This proactive approach minimized waste and boosted profits by aligning their outputs with buyer needs.
Another instance comes from Brazil's coffee industry, where producers used derived demand forecasts to gauge shifts in global coffee consumption. Leveraging data on emerging markets and trend analyses, Brazilian farmers could adapt their production strategies, effectively capitalizing on rising demand from countries like China. The result was an impressive growth in export capabilities, allowing Brazil to maintain its position as a leading coffee supplier.
- Data-Driven Decisions: Successful implementations often feature the integration of various data sources to inform decisions, leading to more accurate forecasts.
- Market Responsiveness: Regions that quickly adapt to market changes tend to see a more favorable economic outcome.
- Collaboration: Partnerships between farmers and agricultural economists enhance the effectiveness of derived demand forecasting, fostering an environment for shared insights.
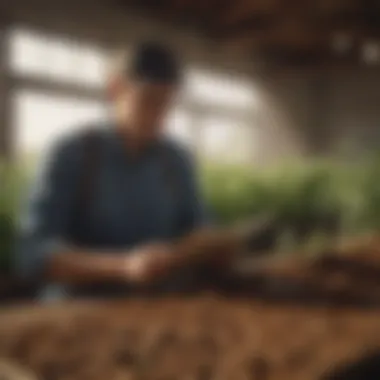
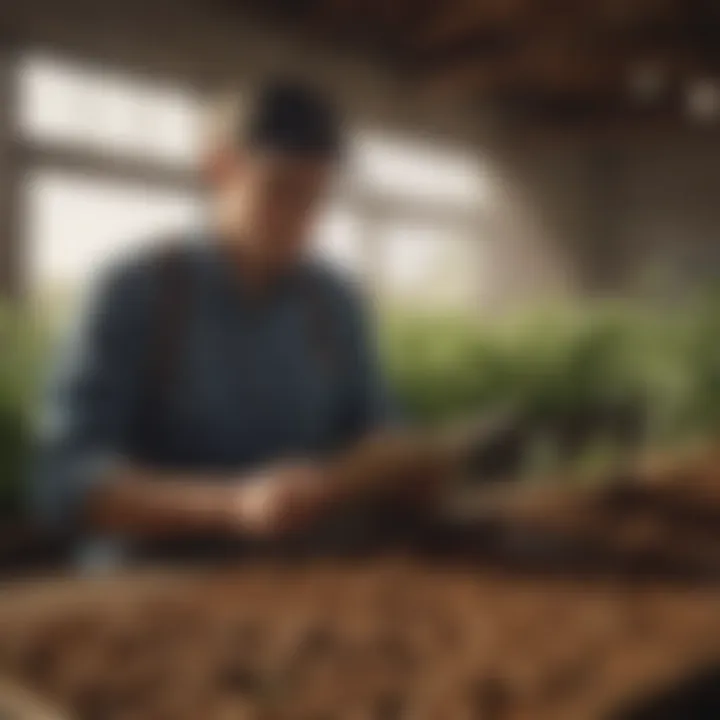
Lessons Learned from Failures
While the case studies above illustrate successful outcomes, not all attempts at derived demand forecasting have yielded positive results. Lessons gleaned from these failures are just as crucial in shaping effective forecasting techniques. A significant reason behind some of these failures can be traced to inadequate data or poor data interpretation, leading to misguided predictions and strategic missteps.
For instance, in a region of Southern Africa, an attempt to forecast maize demand based solely on past yield without considering current market trends resulted in unpleasant surprises for farmers. The failure to account for shifts in consumer preferences and external economic factors left many producers grappling with oversupply and losses.
Another lesson stems from the coffee market's fluctuations in Colombia, where a reliance on outdated data — essentially data from the previous decade — resulted in misallocation of resources. Farmers continued planting in the same way, oblivious to the shifting dynamics of both local and international demand due to new dietary trends.
"Forecasting is like navigating a ship: without a proper compass, you may end up at the wrong destination."
To sum up, the consequences of not adapting derived demand forecasting methods to current realities are evident. Failed case studies serve as stark reminders that it’s essential for farmers and stakeholders to embrace evolving methodologies, integrating fresh data and being responsive to market shifts. This vigilance can help ensure that forecasting remains both relevant and effective in navigating the unpredictable landscape of agriculture.
Challenges in Derived Demand Forecasting
When diving into the world of derived demand forecasting in agriculture, it’s hard to ignore the bumps along the road. Understanding these challenges is essential, as they can significantly shape the accuracy and efficacy of forecasting efforts for farmers and agribusinesses alike. The derived demand method is founded on the premise that the demand for agricultural outputs is inherently tied to various external and internal factors. However, a few hurdles often emerge that can cloud this relationship.
Data Limitations
Data is king in the realm of forecasting. Without it, predictions can flop like a fish out of water. In agricultural forecasting, the need for timely and accurate data is paramount. Yet, many times, this data is simply not available or isn't of the best quality. Here are a few aspects of data limitations that can impact derived demand forecasting:
- Inconsistent Data Sources: Relying on multiple sources for data can lead to inconsistencies. For instance, farmer surveys might yield different results than government reports, leading to confusion in demand assessments.
- Lagging Data: Agricultural data often comes in with a time lag. By the time data reflects current market conditions, the landscape may have already shifted, leaving forecasts outdated.
- Granularity of Data: Surveys may lack specificity, combining various geographical regions or types of crops. This can mask local demand patterns that are crucial for accurate predictions.
Market Volatility
Market volatility can be likened to a roller coaster ride; it often brings unexpected ups and downs that can catch even seasoned farmers off guard. The agricultural market is subjected to various forces, such as geopolitical tensions, climatic variations, and changing consumer preferences. These factors can introduce significant fluctuations that complicate derived demand forecasting:
- Price Swings: Prices for agricultural products can have wild fluctuations based on supply chain factors. For instance, a sudden drought may drastically reduce yield, causing prices to spike unexpectedly just when farmers need to predict demand.
- Competition: The entrance of new players or the exit of established ones can reshape the market landscape overnight. Such changes can make previous demand forecasts obsolete.
- Consumer Behavior Shifts: As societal trends evolve—like a growing thirst for organic products—demand patterns shift. Forecasts based on past consumer behavior may falter in this new reality.
"With the market as unpredictable as the weather, staying ahead of the trends is key for effective derived demand forecasting."
To sum up, market volatility remains a crucial element in the derived demand forecasting equation. It requires constant monitoring and a willingness to adjust strategies on the fly. Being agile in response to market changes will empower farmers and agribusinesses to stay relevant in the face of daunting unpredictability.
Future Trends in Derived Demand Forecasting
As the landscape of agriculture continues to evolve, understanding Future Trends in Derived Demand Forecasting is crucial for all stakeholders involved. This section examines the ongoing shifts that could significantly impact the effectiveness and application of forecasting methodologies in agriculture. Markedly, the rise of technology and changing consumer behaviors are essential angling points, providing insights that could necessitate adjustments in traditional forecasting models. Together, these trends not only help address current challenges but also carve pathways for sustainable practices that benefit both farmers and the environment.
Integration of Artificial Intelligence
Artificial Intelligence (AI) is an undeniable game-changer in the realm of derived demand forecasting. Imagine being able to predict market dynamics with unusual accuracy based on vast amounts of data. AI algorithms can analyze historical data and present trends far more efficiently than human analysts. For instance, machine learning models can be trained to process agricultural yield data alongside consumer purchasing trends, providing farmers with a clearer picture of what crops are likely to be in demand and when.
- Benefits of AI Integration
- Improved Data Analysis: AI tools can systematically assess variables, adjusting for market volatility and other external influences.
- Predictive Capabilities: With enhanced predictive analytics, farmers can make informed choices about crop planning and resource allocation, ultimately reducing waste and maximizing profits.
- Real-Time Insights: Automated systems analyze data in real time, allowing farmers to adapt quickly to changing conditions.
However, there's a caveat. While AI brings substantial advantages, it also requires a certain level of technological investment and skill. Small-scale farmers may find this barrier significant, potentially widening the gap between larger agribusinesses and smaller operations.
"Adapting to AI technologies might not just be a trend; it could be a necessity for survival in modern agriculture."
Emphasis on Sustainability
In recent years, an increased emphasis on sustainability has become not merely a trend, but a vital component of derived demand forecasting. With climate change and environmental concerns on everyone’s mind, forecasting models are evolving to incorporate sustainable agricultural practices. This approach considers not only the demand for products but also the ecological impacts of production methods.
- Sustainability Considerations
- Resource Management: By focusing on sustainable practices, farmers can forecast demand while also considering the long-term health of their land.
- Consumer Preferences: As more consumers prefer sustainably sourced foods, forecasting that integrates this preference can lead to better market alignment.
- Policy Implications: Upcoming policies may further tilt the scales in favor of sustainable practices, prompting forecasts to pivot accordingly.
The integration of sustainability into derived demand forecasting enhances the relevance and applicability of such models in today’s market. It represents a holistic view of agricultural planning, retaining profitability without sacrificing environmental integrity. Farmers and producers who grasp these trends will likely be better positioned to meet both market demands and societal expectations in the coming years.
The End
In wrapping things up, the derived demand method of forecasting in agriculture holds critical significance for both the immediate and long-term functioning of the agricultural sector. Understanding this method allows stakeholders to make informed decisions that can enhance productivity and profitability. This article has highlighted several specific elements, benefits, and considerations regarding the application of this method.
Summary of Key Insights
- Foundation of Demand: The underpinnings of derived demand lie in recognizing how the demand for agricultural outputs is intricately linked to consumer preferences, technology, and wider economic trends. Knowing how these factors interact equips producers to tailor their offerings effectively.
- Predictive Power: Through various forecasting techniques, derived demand provides an avenue for predicting future trends in agriculture. Techniques like quantitative modeling offer numbers and forecasts, while qualitative methods consider the socio-cultural landscape. Both are essenital in creating a comprehensive forecasting model.
- Examples from the Field: The case studies reviewed have shown tangible results from employing derived demand forecasting in regions around the world. Successful implementations often highlight how farmers adapted their strategies, leading to remarkable increases in yield and revenue.
- Challenges and Adaptations: It is clear that obstacles, such as data limitations and market volatility, still exist. Understanding these challenges not only prepares stakeholders but also enhances their strategies toward mitigation.
Implications for Stakeholders
The implications of derived demand forecasting extend far beyond mere numbers. For farmers, its insights can drive decisions about what crops to plant—not only for this season but also for the years ahead. Agronomists can use these insights to adapt practices for sustainability, while agricultural economists benefit from nuanced understandings of market trends.
- Decision-Making Framework: Stakeholders can create action plans based on forecasts, ultimately leading to resource optimization.
- Collaboration Opportunities: Knowledge-sharing among different actors in agriculture can enhance efforts; when farmers talk to agronomists, the integration of new insights can amplify productivity.
- Policy Formulation: For policymakers, understanding derived demand dynamics can lead to more informed regulations and support mechanisms that foster resilience in agricultural sectors.
In sum, the derived demand method of forecasting in agriculture opens a treasure trove of insights and possibilities. Stakeholders who tap into its potential will likely navigate the complex agricultural landscape more effectively, ensuring a robust and sustainable future.